Understanding the Role of Labeling Tool Machine Learning in Today's Business
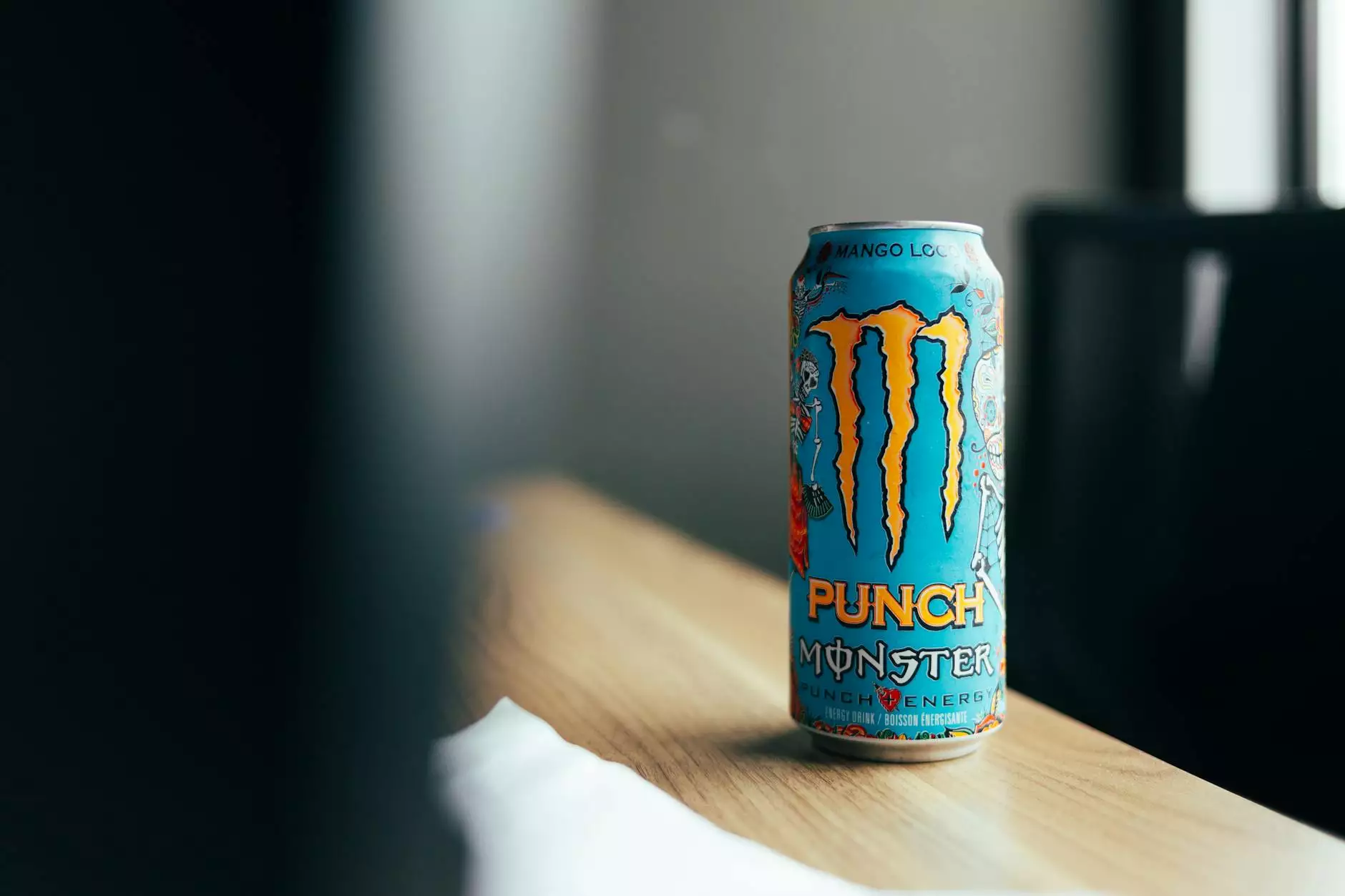
In the age of data, businesses are increasingly reliant on advanced technologies to help them extract valuable insights from the vast amounts of information they collect. One of the most pivotal tools in this technological arsenal is the labeling tool machine learning. This article explores the significance of this tool in the context of data annotation and its transformative effects on business operations.
The Importance of Data Annotation in Machine Learning
Data annotation involves the process of labeling data so that machine learning models can recognize and understand it. It's a foundational step in developing algorithms that can perform tasks such as image recognition, sentiment analysis, and more. Without properly annotated data, even the most sophisticated machine learning models will struggle to function effectively.
Why Is Data Annotation Essential?
- Quality Output: Annotated data ensures that machine learning models can learn from precise examples, leading to more accurate predictions and classifications.
- Training Efficiency: Well-labeled datasets accelerate the training process, allowing businesses to deploy their models faster.
- Model Adaptability: Comprehensive data annotation helps models adapt to various scenarios, making them more robust and versatile.
Labeling Tool Machine Learning: An Overview
The labeling tool machine learning leverages automation and artificial intelligence to streamline the data annotation process. These tools can significantly reduce the time and effort required to label massive datasets, which is a common obstacle for many businesses.
Benefits of Using Labeling Tools
- Increased Accuracy: Automated labeling tools minimize human error, ensuring that the data is consistently labeled.
- Cost-Effectiveness: By reducing the time spent on manual annotation, businesses can cut costs associated with data preparation.
- Scalability: These tools allow businesses to scale their data operations efficiently, handling larger datasets without a proportional increase in resource allocation.
Key Features of High-End Labeling Tools
When selecting a labeling tool, it's crucial to consider certain features that set the most effective tools apart. A top-tier labeling tool machine learning should offer:
1. User-Friendly Interface
An intuitive interface allows users to navigate the labeling process seamlessly, reducing the learning curve and increasing productivity.
2. Customization Options
High-quality tools provide customizable labeling interfaces to adapt to specific project needs and data types.
3. Integration Capabilities
The best tools integrate easily with existing systems and platforms, ensuring a smooth workflow without significant disruptions.
4. Support for Various Data Types
From images to text and audio, a robust labeling tool must support multiple data formats to cater to diverse machine learning projects.
5. Collaboration Features
Collaboration tools enable teams to work together efficiently, keeping all annotators on the same page and streamlining the review process.
Real-World Applications of Labeling Tool Machine Learning
Businesses across various sectors utilize labeling tool machine learning to enhance their operations:
Healthcare
In healthcare, accurate data annotation helps in tasks such as disease diagnosis and patient monitoring through image analysis. Annotated medical images allow AI models to identify illnesses with greater accuracy.
Retail and E-commerce
Retail businesses use machine learning for customer segmentation and personalized marketing. Labeling customer data enables better recommendations and improves customer satisfaction.
Automotive Industry
Self-driving cars rely heavily on machine learning for safe navigation. High-quality image labeling tools are critical for teaching these systems to interpret their surroundings accurately.
Finance
In finance, labeled transactions help detect fraudulent activity. Machine learning models trained on labeled datasets can spot anomalies and protect businesses from financial loss.
Choosing the Right Labeling Tool Machine Learning
With numerous options available, selecting the best labeling tool machine learning is essential for optimizing your data processes. Consider the following steps:
1. Assess Your Needs
Evaluate the specific requirements of your projects, including the types of data you are working with and the scale of your labeling tasks.
2. Research Available Tools
Investigate various tools in the market, comparing their features, pricing, and user reviews to identify those that meet your criteria.
3. Test with Demos
Many software providers offer demos or trial periods. Take advantage of these to test usability and performance in your environment.
4. Evaluate Support and Documentation
Strong customer support and comprehensive documentation can be invaluable, especially during the initial setup and integration phases.
Future Trends in Labeling Tool Machine Learning
As the demand for data annotation grows, the field of labeling tools is undergoing significant advancements. Here are some trends to watch:
1. Increased Automation
The rise of automated labeling processes powered by AI will continue to improve efficiency and reduce the reliance on manual input.
2. Enhanced Collaboration
Tools that facilitate team collaboration will become increasingly vital, enabling annotations to be performed faster and with better accuracy.
3. Better User Experience
Ongoing focus on user experience will lead to the development of more intuitive and accessible platforms, empowering users of all skill levels to engage in data annotation effectively.
4. Advanced Quality Control Features
Quality assurance processes will evolve, with tools offering more sophisticated capabilities to ensure data accuracy and reliability before training machine learning models.
The Competitive Edge of Keylabs.ai
At keylabs.ai, we understand that the quality of your data annotation can make or break your machine learning projects. Our data annotation tools and data annotation platform are designed with the latest advancements in mind, providing businesses with a cutting-edge solution to their labeling challenges.
By leveraging our state-of-the-art technology, businesses can achieve:
- Superior Data Quality: Enhance the accuracy of your models with well-annotated datasets.
- Streamlined Processes: Reduce time-to-market for your data-driven applications.
- Scalability: Handle growing data demands without compromising on quality.
Conclusion
In conclusion, the evolution of the labeling tool machine learning is driving significant changes in how businesses approach data annotation. By adopting advanced labeling tools like those provided by keylabs.ai, organizations can not only enhance the accuracy and efficiency of their data processes but also gain a competitive edge in their respective industries.
Embrace the power of machine learning today, and watch as your business transforms through informed decision-making and data-driven strategies.