Maximizing the Potential of Image Datasets for Classification
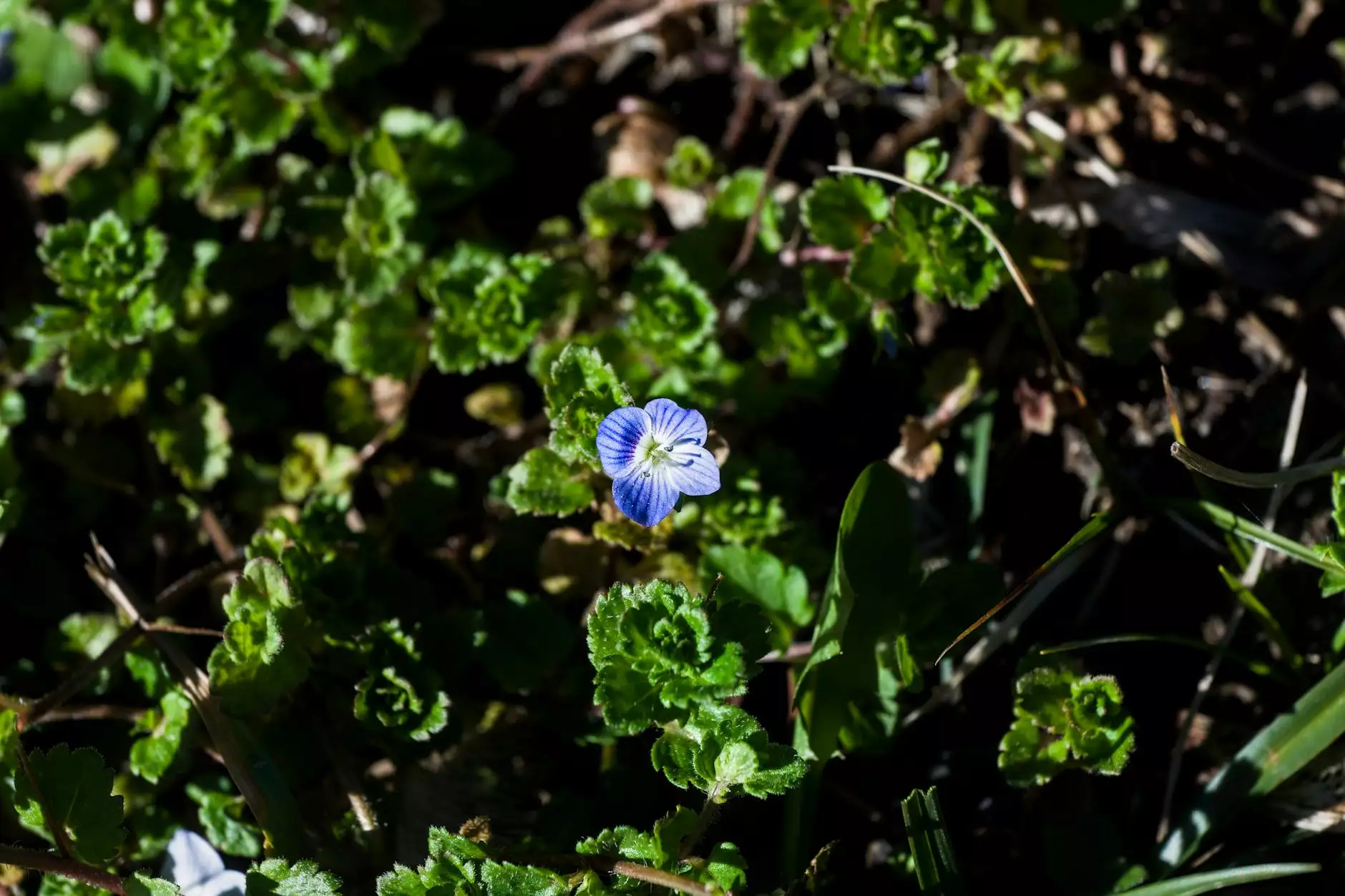
Image datasets for classification have become vital assets in the realm of machine learning and artificial intelligence. As businesses increasingly rely on data-driven decisions, understanding how to effectively utilize these datasets is crucial for success. This article outlines the significance of image datasets, their role in classification tasks, and how high-quality data annotation tools and platforms can streamline the process.
Understanding Image Datasets
In the world of machine learning, an image dataset is a collection of images organized for the purpose of training algorithms to recognize patterns and make predictions. These datasets serve multiple applications, including:
- Object recognition
- Facial recognition
- Medical image analysis
- Automated driving systems
- Content moderation
Each image in a dataset can be associated with specific labels or tags that aid these algorithms in understanding the content present in the images, thereby enhancing their ability to classify and interpret visual inputs effectively.
The Importance of Quality in Image Datasets for Classification
The effectiveness of machine learning models largely hinges on the quality of the image datasets used during their training. Factors that contribute to dataset quality include:
- Diversity: A wide-ranging set of images that accounts for various angles, conditions, and perspectives increases a model's ability to generalize.
- Label Accuracy: Precise labeling is essential for the correct classification of images. Any discrepancies can lead to significant errors in model predictions.
- Size: Larger datasets often contribute to better model performance, provided the quality is maintained. A robust dataset minimizes the chances of overfitting.
- Relevance: The dataset must be relevant to the intended application. For instance, medical imaging models should train on images specifically tailored for medical diagnostics.
Utilizing Data Annotation Tools
To construct high-quality image datasets for classification, businesses require effective data annotation tools. These tools facilitate the process of labeling images accurately, enabling machine learning models to receive precise training. At Keylabs.ai, we offer a variety of advanced data annotation solutions that cater to different industry needs, such as:
- Image Segmentation: Dividing an image into segments to simplify its analysis.
- Bounding Box Annotation: Encapsulating an object within a rectangular box to assist with object detection.
- Polygon Annotation: Drawing polygons around complex objects for accurate classification.
- 3D Point Cloud Annotation: Annotating 3D data points for comprehensive analysis in sectors like automotive and robotics.
By leveraging these tools, organizations can create datasets that empower their machine learning models to achieve optimal performance.
The Role of a Data Annotation Platform
A robust data annotation platform is central to the success of any machine learning project. The following features are crucial when choosing a data annotation platform:
1. Scalability
The platform must be capable of handling large volumes of data efficiently, allowing for the swift addition and annotation of images.
2. Collaboration
Enhancing teamwork and streamlining processes among data scientists, annotators, and stakeholders is essential. Look for platforms that promote collaborative annotation and feedback mechanisms.
3. Customization
A good platform will allow businesses to customize their annotation templates and processes according to their specific needs and industry standards.
4. Integrations
Integrating with existing workflows, data management systems, and machine learning frameworks provides a seamless experience for users and helps maintain consistency across projects.
5. Quality Control Features
Incorporating features such as automated quality checks enhances the reliability of the annotations, reducing errors that could hinder model performance.
Best Practices for Working with Image Datasets for Classification
To fully harness the potential of image datasets, businesses should adhere to several best practices:
1. Start with a Clear ObjectiveDefine what you want to achieve with your classification model. Knowing the end goal will guide your dataset creation and annotation processes.
2. Preserve Data IntegrityMaintaining the integrity of your dataset is vital. Ensure that your images remain unchanged during the annotation process, as any modifications can introduce bias and inaccuracies.
3. Test and ValidateOnce your model is trained, it’s essential to validate its performance against a separate test set. Continuous testing helps identify weaknesses in the model and allows for iterative improvements.
4. Update RegularlyAs new data becomes available, periodic updates to your datasets will keep your models relevant and capable of adapting to new trends and challenges.
5. Focus on Noise ReductionHigh-quality image datasets should be free of irrelevant data or 'noise.' As such, regular cleaning of the dataset can enhance the performance of your classification algorithms.
Case Studies: Successful Uses of Image Datasets for Classification
Many organizations have benefitted from utilizing image datasets effectively. Below are a few case studies demonstrating their impact:
Case Study 1: Healthcare Diagnostics
A leading medical imaging firm developed a classification model to assist radiologists in detecting tumors from X-ray images. By utilizing a richly annotated dataset of thousands of images, the model achieved remarkable accuracy, leading to faster and more reliable diagnoses.
Case Study 2: Autonomous Vehicles
Automotive companies have successfully employed image datasets for the classification of road signs and pedestrian recognition. By annotating millions of images gathered from diverse driving conditions, these firms have advanced the development of safer autonomous driving systems.
Case Study 3: E-commerce
Online retail platforms have utilized image datasets for classification to enhance their recommendation systems. By accurately tagging product images, these platforms analyze consumer behavior to recommend similar items, improving customer satisfaction and sales.
The Future of Image Datasets for Classification
The future of image datasets for classification looks promising, with advancements in technology driving new possibilities. Innovations such as generative adversarial networks (GANs) and transfer learning are shaping the way we create and use datasets. Furthermore, the incorporation of artificial intelligence in data annotation processes promises increased efficiency and accuracy. As businesses continue to navigate the digital landscape, investing in high-quality image datasets and annotation tools will remain essential for staying competitive.
Conclusion
In conclusion, the power of image datasets for classification is undeniable. Businesses must focus on quality, relevance, and effective annotation to unlock the full potential of their machine learning projects. By leveraging sophisticated data annotation tools and platforms, such as those offered by Keylabs.ai, organizations can enhance the accuracy of their models, leading to improved decision-making and outcomes.
As the demand for accurate and reliable machine learning applications grows, so too will the importance of high-quality image datasets. Organizations that prioritize the construction and maintenance of these datasets will position themselves at the forefront of innovation and success in their respective industries.